Artificial intelligence was a topic of focus for the medical device industry in 2024.
Companies including GE Healthcare, Medtronic and Dexcom touted new AI features, and others like Stryker and Quest Diagnostics added AI assets through M&A. Meanwhile, conversations about regulations and generative AI, models that are trained to create new data including images and text, dominated medtech conferences.
The Food and Drug Administration recently clarified what information it wants to see in future submissions as the number of AI-enabled devices authorized by the agency has eclipsed 1,000. The FDA still has not authorized any tools that continuously adapt or use generative AI.
While the guidance documents should provide some clarity for medical device developers, questions still loom about how regulators will approach generative AI. The Trump administration also brings uncertainty about how AI will be regulated.
The technology still faces barriers to adoption, including a lack of insurance reimbursement, despite all the buzz AI has garnered in the medtech industry.
MedTech Dive interviewed experts about AI trends they’re watching in 2025. Here are their predictions:
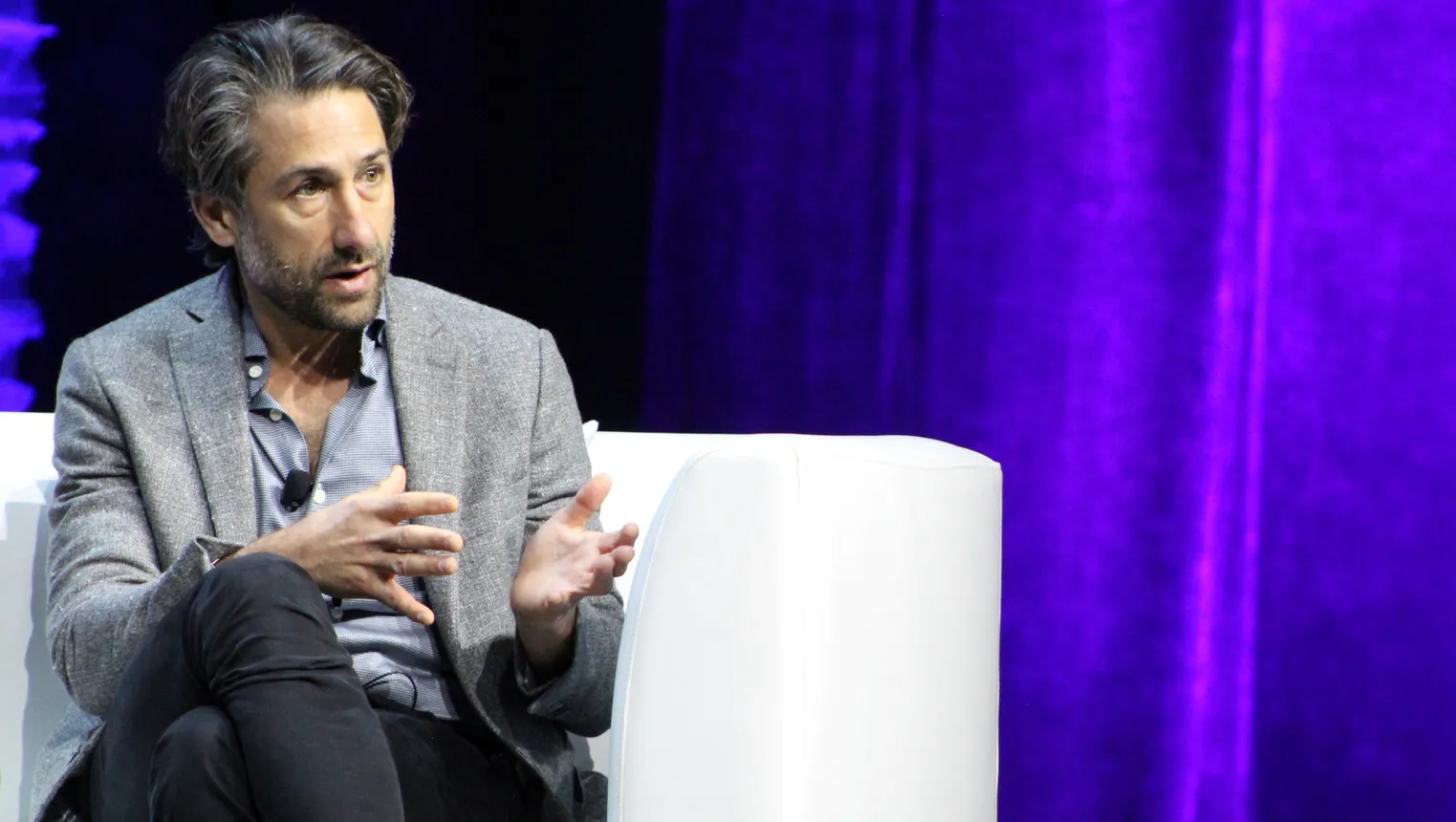
1. New AI guidances bring clarity to device developers
Attorneys said the FDA’s recent guidances on AI-enabled devices should provide more clarity for developers.
In December, the agency finalized guidance on pre-determined change control plans (PCCPs), a new framework that allows for pre-specified modifications to devices after they are on the market.
Amanda Johnston, a partner at Gardner, an FDA-focused law firm, expects more companies to submit PCCPs and for the FDA to emphasize this new approach.
“I see some of that being a request on the FDA’s end,” Johnston said. “I do think that they will try to push developers into that framework.”
PCCPs require more work upfront for developers, but they can save time and money on postmarket submissions with careful planning, Johnston added.
In January, the FDA issued draft guidance outlining what information the agency wants to see in submissions for AI devices and when postmarket monitoring might be needed. The draft also encourages developers to consider PCCPs.
Megan Robertson, an attorney with Washington, D.C.-based law firm Epstein Becker Green, said the latest draft guidance is one developers should “keep in your back pocket” and use like a checklist when putting together submissions.
Robertson expects to see more submissions for AI-enabled devices as companies become more familiar with the FDA’s approach. Many products in the agency’s breakthrough device program involve software or AI components, she added.
It’s not clear how President Donald Trump’s new administration will approach AI. On his first day in office, Trump rescinded a sweeping executive order on AI signed by former President Joe Biden that had called for the Department of Health and Human Services to form an AI task force. Earlier this month, the HHS released a strategic plan for overseeing AI in healthcare in response to the executive order.
Martin Makary, whom Trump nominated for FDA commissioner, has not spoken much on the subject. Makary shared a JAMA article written last year by Scott Gottlieb calling for Congress to update FDA regulations on medical AI.
“You can't really compare the first [Trump] administration to this one to necessarily make any concrete predictions,” Robertson said. “But we do think it's likely that this administration could take action to roll back some of the more limiting or controversial industry actions that FDA took during the Biden administration, like the clinical decision support final guidance.”
The FDA’s 2022 final guidance clarified when certain software functions should be regulated as devices. Epstein Becker Green filed a petition on behalf of the Clinical Decision Support Coalition in 2023 for the FDA to rescind the guidance, saying it unnecessarily increases the regulatory burden for developers.
Johnston expects AI and machine learning will continue to be a focus for the agency under the Trump administration. The attorney also flagged a growing patchwork of state and national privacy laws that could affect AI adoption as a topic to watch.
2. Payment challenges remain
AI features can be incorporated into medical equipment, such as imaging machines, or sold as standalone software platforms. A challenge for device companies has been figuring out how to price these features, given that insurance does not cover them.
Currently, the Centers for Medicare and Medicaid Services does not provide specific reimbursement for FDA-authorized AI technology, said BTIG analyst Ryan Zimmerman. Companies have to use Medicare’s New Technology Add-on Payments pathway, or another workaround to get covered, Zimmerman added.
Last year, a bipartisan group of senators wrote a letter to the CMS calling for a payment pathway for algorithm-based healthcare services. A House task force report later in 2024 found that “CMS has allowed for limited Medicare coverage of AI technologies” when the services meet coverage criteria.
Companies are pitching hospitals on AI features to speed up processes and reduce staffing pressures, Zimmerman said. However, those customers are more closely scrutinizing AI tools to see if they’re worth the cost, said Brian Anderson, CEO of the nonprofit Coalition for Health AI (CHAI).
After a “lot of excitement” around AI last year, “now we're seeing a little bit of a sobering perspective that if we're going to be spending a large amount of capital to purchase these things, that we need to make sure that we're seeing economic return on investment,” Anderson said. “I’m hearing health systems demanding that of vendors more.”
3. More focus on foundation models and administrative tools
Most of the AI tools regulated today by the FDA are in radiology, although more are being used in pathology, ophthalmology and cardiology. A growing number of companies are also using large language models for administrative tasks, such as generating clinical notes. Firms including GE Healthcare are developing other types of foundation models, which are large-scale models that can be used for a variety of purposes, such as processing MRI images, pulling information from doctor’s notes or analyzing electronic health record data.
Nina Kottler, associate chief medical officer of clinical AI for Radiology Partners, said AI solutions used in radiology have “absolutely changed” in the last decade.
Initially, AI tools were focused on detecting or triaging for a specific condition, such as software that analyzes images to detect potential stroke cases. Now, more AI solutions are focused on workflow, Kottler said.
“This gap between volume and capacity has been growing for years,” Kottler said, adding that the mismatch “is so great that it takes over any other use case you’ll consider.”
Kottler is paying attention to two types of foundation models. One is language models that can summarize words into a report. These features are already being sold, such as a tool made by Rad AI to generate radiology report impressions from the findings and clinical indication. These text-based models currently aren’t regulated as medical devices.
“There's a question of whether that will be true in the future, but for now, it is excluded,” Kottler said.
Kottler is also watching vision language models that can analyze an image and then craft a draft report. These would fall under device regulations. Companies started building and testing these types of models last year, but none have been authorized by the FDA, Kottler added.
Epstein Becker Green’s Robertson said developers that want to make a submission for a medical device that uses generative AI may have more resources to develop a regulatory strategy than they did last year. However, it remains to be seen how the FDA, under a new administration, will perceive risks with generative AI models.
"At the end of the day, the software, while it may not be doing diagnosis directly, is a big part of what a physician would use to come out with a diagnosis."

Francisco Rodríguez Campos
Principal project officer at ECRI
Francisco Rodríguez Campos, principal project officer at the patient safety group ECRI, said that while some AI tools may only be used for administrative purposes, hospitals should still treat them with the same scrutiny as other AI devices.
“I have seen so many issues,” Rodríguez Campos said, adding that one hospital using a note generation tool found that after updating to the latest version, the tool didn’t work as well.
“At the end of the day, the software, while it may not be doing diagnosis directly, is a big part of what a physician would use to come out with a diagnosis,” Rodríguez Campos said. “They are having an influence in the delivery of health.”
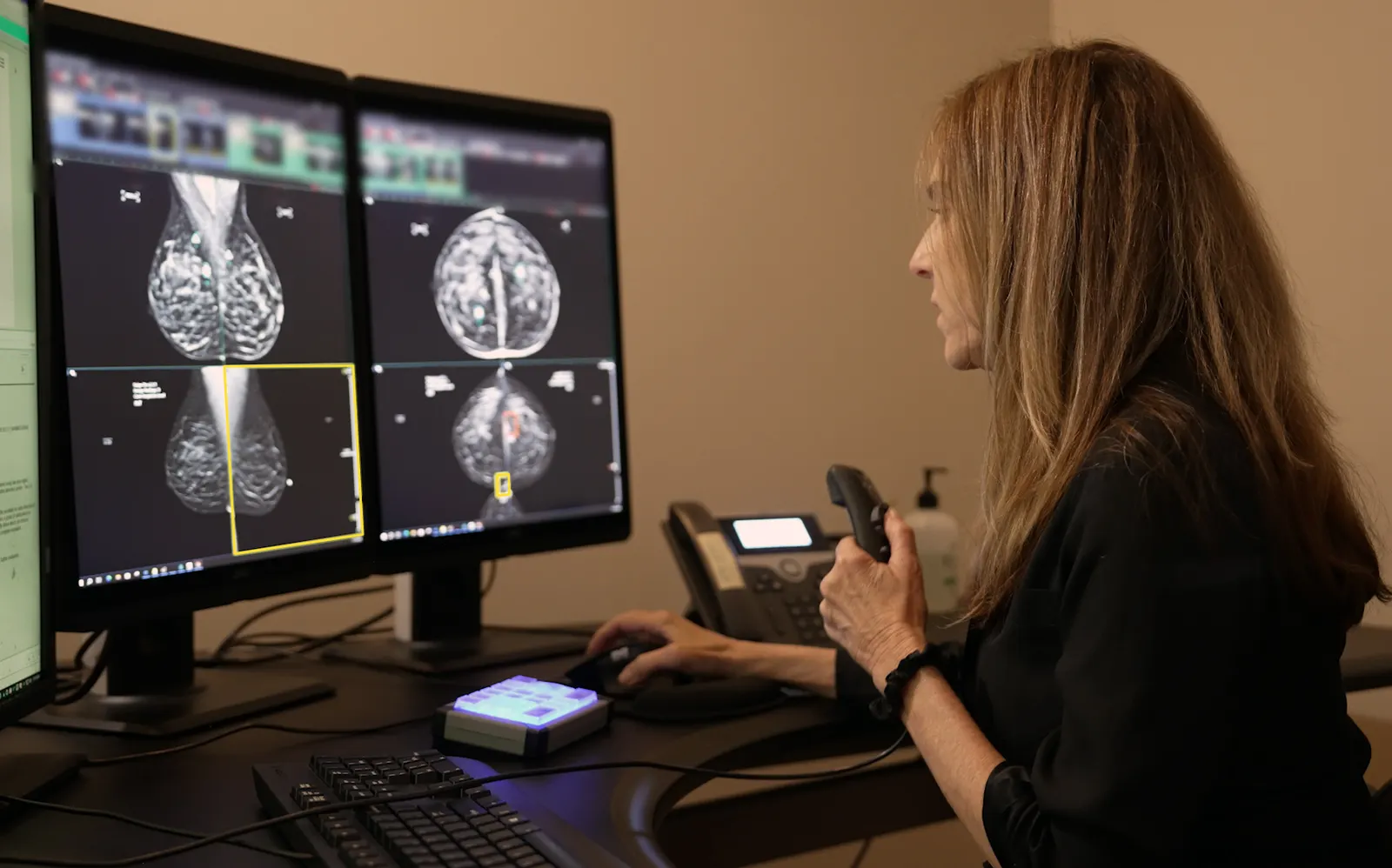
4. Hospitals need more information to evaluate AI tools
As AI devices become more prominent, so do questions about governance, such as who is responsible for maintaining models and ensuring they work as intended. Experts said hospitals need more information before a purchase is made and support afterward for performance monitoring.
Scott Lucas, ECRI’s vice president of device safety, raised concerns about a confluence of factors: The hype, the promise and the rapid evolution of AI tools, and an environment where there are “far too many preventable incidents” in healthcare.
Whether hospitals have AI solutions, or can monitor and govern them, can “range quite a bit depending on the resources of the facility,” Lucas said.
A recent study in Health Affairs found that only 61% of hospitals that use predictive models tested them on their own data, and fewer evaluated the models for bias. The article found hospitals that were part of a larger system and had the highest operating margins were most likely to evaluate models locally.
Evaluating AI models on local data is important because the performance data developers share with the FDA don’t always generalize well across different practices, said Radiology Partners’ Kottler.
“It doesn't necessarily reflect that it's going to work well on my data,” Kottler said.
Radiology Partners goes through five steps for evaluating AI models before deciding to roll them out. The practice has used this process for computer vision models that analyze images, as well as large language models that populate notes, Kottler said.
“You could have a very accurate model, but if it's only finding the same things that the radiologist finds, it's actually not that helpful. You’re just paying money for something that you’re already paying money for.”

Nina Kottler
Associate chief medical officer of clinical AI for Radiology Partners
First, Radiology Partners looks at a model’s performance on its own data, ideally with a large number of cases and with natural disease prevalence. It also looks at whether a model picks up cases that radiologists would have otherwise missed.
“You could have a very accurate model, but if it's only finding the same things that the radiologist finds, it's actually not that helpful,” Kottler said. “You’re just paying money for something that you’re already paying money for.”
Kottler also looks for “wow” cases, that would be impressive for radiologists, and for pitfalls or false positives, so that radiologists know what types of errors a model is likely to make. Then, they summarize their findings and decide.
Groups like CHAI have also advocated for tools intended to provide more upfront information. For example, CHAI has suggested using model cards, which Anderson described as a “nutrition label” that provides details such as how an AI model is trained and what datasets were used. The FDA referenced model cards as a transparency tool in its January draft guidance.
CHAI has also been building a network of assurance labs, or third-party labs that can evaluate a model objectively across populations that would be representative of patients seen at a health system, for a “more informed procurement process,” Anderson said.
After hospitals start using an AI tool, they also need to monitor it over time to ensure the performance doesn’t degrade. That involves collaborating with a vendor early on, the CEO added.
“These health systems didn't appreciate … how challenging that would be, how expensive it would be, and how important [it is] to have a strong partnership with the vendors,” Anderson said. “Monitoring these models is not something you can do on your own, and you need that partnership.”